Bad data gets in the way of making smart choices. For businesses, quality information is key for good decision-making, much like how an organized drawer makes it easy to locate everything you need.
This is where data cleansing saves the day. With duplicate records removed, formats standardized, and errors corrected, companies can tap into the full power of data analytics. Without data cleansing, the potential of all that business data stays locked up. But, with clean databases, enterprises can uncover game-changing revelations and opportunities.
Why do businesses need to clean their databases?
Data cleansing, also known as data scrubbing or data cleaning, is the initial phase of data preparation. It involves identifying and rectifying or removing incorrect, incomplete, inaccurate, or irrelevant data from the dataset. This process can be performed manually or assisted by specialized software.
Data cleansing serves as the foundation for data quality and integrity, ensuring that businesses can trust and rely on the insights derived from their datasets.
The significance of data cleansing cannot be overstated. As companies become increasingly dependent on data to make critical decisions, having a “clean” database becomes a top priority. Inferior data quality can lead to misinformation, misguided strategies, and faulty conclusions, hampering the overall success of an organization.
Common types of data problems
Duplicate data: Occurs when there are two or more identical records in the dataset. It can lead to skewed analyses, inaccurate metrics, and wasted resources.
Conflicting data: Arises when conflicting information is present within the same record. For example, a customer may have different phone numbers or addresses in different records. Resolving conflicts ensures consistency and reliability in data-driven decisions.
Incomplete data: Refers to data that contains missing attributes or fields. Incomplete data can render analyses inconclusive and prevent organizations from getting a comprehensive view of their operations.
Invalid data: Represents data that does not adhere to predefined standards. Invalid data can arise due to data entry errors or the absence of validation checks, undermining the credibility of the entire dataset.
The dangers of “Bad Data”
Poor-quality data can significantly impact a company’s bottom line. Studies show that poor data quality impacts the revenue of 88% of US companies. In the United States alone, dirty data incurs an approximate annual loss of $3.1 trillion.
Moreover, bad data consumes a considerable portion of data analysts’ time, leading to decreased productivity. Ineffective marketing campaigns and unreliable customer information are also among the adverse effects of bad data.
It affects various functions within an organization, hindering strategic planning, market analysis, customer relationship management, and financial reporting. The consequences of basing decisions on faulty data can be dire, resulting in lost opportunities, decreased competitiveness, and compromised customer experiences.
Some benefits of data cleansing
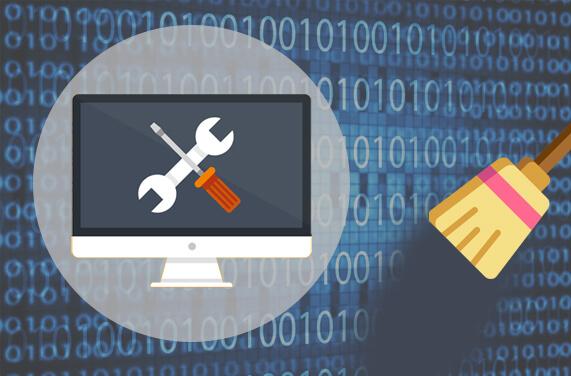
- More accurate insights and reliable predictions
Cleaning data leads to verified information, enabling businesses to make accurate predictions and informed decisions across various domains. With such a dependable dataset, companies can confidently explore trends and patterns, facilitating better forecasting and risk assessment.
- Increased productivity and effectiveness
Eliminating data bottlenecks enhances employees’ efficiency and effectiveness in their roles. Data analysts and other stakeholders can focus on value-added tasks rather than correcting errors in the dataset they are supposed to analyze.
- Decreased overall cost and increased revenue
Proper data cleansing minimizes revenue losses caused by poor data quality, positively impacting the company’s financial performance. By avoiding the time-investment and costs associated with data-related errors and inefficiencies, organizations can utilize their resources more effectively.
- Enhanced customer satisfaction
Accurate data allows businesses to better understand their customers and design their experiences more effectively. By leveraging clean data, companies can personalize their offerings, address customer pain points, and build long-lasting relationships.
Data Cleansing Best Practices
- Develop a data quality strategy:
- Clearly define data quality requirements and align them with business goals.
- Establish measurable metrics to evaluate data quality and track improvements.
- Analyze historical data to identify patterns of inaccuracies or inconsistencies.
- Investigate the reasons behind data issues and implement preventive measures.
- Implement procedures for ongoing data validation and cleaning.
- Correct data at the point of entry
- Establish guidelines for data entry to maintain a clean and standardized database.
- Implement automated validation checks to prevent incorrect data from entering the system.
- Promote a data-driven culture within the organization, where data quality is everyone’s responsibility.
- Validate the accuracy of your data
- Leverage data quality software and tools to validate and cleanse data efficiently.
- Regularly assess data quality and identify areas for improvement.
- Involve data analysts and subject matter experts in data validation efforts to ensure accuracy and completeness.
- Manage duplicates
- Implement data cleansing routines to identify and remove duplicates during data entry or data integration processes.
- Apply appropriate data transformation techniques (standardizing, normalizing, merging, aggregating, filtering, scaling, and removing outlier data points) to maintain a consistent and accurate dataset.
- Append missing data
- Partner with reputable data providers to fill in missing information and enhance data completeness.
- Use data enrichment techniques to update and complete existing data records.
- Promote the use of clean data across the organization
- Conduct training sessions and awareness campaigns to emphasize the significance of clean data.
- Implement data governance practices to ensure data quality and consistency across the organization.
- Continuously monitor data quality and track improvements over time to foster a data-driven culture.
In conclusion
The companies that will lead their industries tomorrow are those who decide to prioritize the health of their data today. That’s because comprehensively cleansing business data is a crucial investment that pays long-term dividends. The transformation of once-unreliable data into a strategic asset enables businesses to thrive amidst growing complexity and competition; it gives you the context and confidence to make bold yet calculated decisions.